AI for Pharma
Leverage AI to Accelerate Drug Discovery and Development Efforts
18 - 19 October 2022 ALL TIMES CEST
Tuesday, 18 October
Registration and Morning Coffee (Foyer)07:30
ROOM LOCATION: MOA 9
PLENARY KEYNOTE PROGRAM
PLENARY KEYNOTE PRESENTATION: To Unlock the Power of AI, It’s Time To Stop Thinking Human
Richard Law, PhD, Chief Business Officer, Exscientia
We’ve spent more than a decade hearing about the promise of AI for drug discovery and development. While that promise remains, it’s time to evolve our approach to unlock it. For all the buzz about AI, most companies are still using AI-assisted approaches, where humans focus on leveraging “good data” to fuel AI. Yet AI platforms, at heart, are data agnostic and make “decisions” far beyond human comprehension. No human can think in say, a 2,500-dimensional space. It’s too complicated, requires too much learning, and too much data to be managed by humans. In short, humans are still calling the shots, using AI to problem-solve along the way. To unlock the power of AI for drug development and discovery, it is time to remain patient-centric, but stop thinking like humans and allow platforms to be designed to learn and become increasingly powerful and accurate with each incremental piece of data analyzed. In this talk, we’ll discuss the power of this approach and learn about industry players who are embracing this new “AI First” way of re-engineering drug discovery processes – the leap to full, end-to-end integration of artificial intelligence – to maximize the potential of AI and machine learning to create better medicines faster and smarter.
Grand Opening Coffee Break in the Exhibit Hall with Poster Viewing (Room Location: MOA 11)10:15
ROOM LOCATION: MOA 2
ACCELERATING DRUG DISCOVERY WITH ARTIFICIAL INTELLIGENCE AND MACHINE LEARNING: TECHNOLOGY TRENDS AND SHIFTS
AI Opportunity for Drug Discovery and Development
Unmesh Shankar Lal, Director, Healthcare & Life Sciences, Frost & Sullivan, Inc.
Artificial Intelligence (AI) for drug discovery and development has entered a growth phase. This presentation will focus on the commercial elements and call out major current and emerging applications of AI/ML within the sector. Benchmarking of the AI-enabled competencies of big tech and start-ups will be followed by a brief assessment of the recent trends, growth opportunities and strategic imperatives. An overview of the emerging ecosystem, partnerships, and selective case studies will be discussed. Key insights will be shared including: 1) themes driving AI application across the drug discovery & development value chain; 2) dynamics around regional trends, vendor eco-system, innovative business models and game-changing companies, and 3) investment opportunities and growth potential.

Ioannis Xenarios, PhD, ex-officio CSO, NGS/AI division, JSR Life Science
JSR Life Sciences (JLS) has built integrated capabilities to de-risk and accelerate the discovery and development of new large molecules therapeutics. The NGS/AI Division, a part of JLS, has developed comprehensive omics-based characterization of bio-samples to support innovation in cancer biology and in cell line and gene therapy development. This presentation will describe our computational and experimental work supporting the discovery and validation of novel molecular targets and biomarkers.
Networking Lunch (Room Location: MOA 11)12:55
Dessert Break in the Exhibit Hall with Poster Viewing (Room Location: MOA 11)13:55
APPLICATION OF AI AND MACHINE LEARNING IN EARLY DRUG DISCOVERY
VirCAD: An HPC-Powered AI/ML BioCAD Platform for Viral Bioengineering
Stefan N. Lukianov, PhD, CEO, Technology, Salve Therapeutics, Inc.
Current gene therapies are mostly limited to plasmid-based and adeno-associated virus variants with inefficient response rates and limited use. Better viral delivery methods would expand the available pharmacopeia to produce precision medicines for intractable and incurable genetic diseases. We are building VirCAD (Virus Computer-Aided Design), an HPC-powered, cloud-accessible, AI/ML-capable bioCAD platform to mine, design, model and test new viral drugs for improved cell and gene therapies, vaccines, oncolytics, antibiotics and other categories.
Augmented Drug Design
The pharmaceutical industry is seeking to accelerate drug discovery through a renewed focus on the valuable data it has accumulated and find ways to use these data far more effectively through artificial intelligence and machine learning. Augmented Drug Design is the program driving AstraZeneca’s efforts in the drug design space with the aim of significantly reducing the time to develop candidate drugs. The Augmented Drug Design platform uses centralized data access guided by FAIR data principles, high-performance computational modelling coupled with AI/ML insights, scaled physics-based methods, underpinned by cloud architecture and services to provide our scientists with novel drug design capabilities. Since program inception, these technologies have been deployed against 70% of our small molecule projects and have significantly impacted several areas including molecular ideation, library design decisions, synthetic route planning, patent research and writing and have already contributed to accelerations in the discovery pipeline.
In vivo, in vitro, in silico, and in Machino: A Smooth Technology Shift to AI for Drug Discovery
Parthiban Srinivasan, PhD, Professor, Data Science and Engineering, Indian Institute of Science Education and Research
Drug discovery is experiencing a fundamental shift in terms of evolving from in silico to in machino which refers to novel molecules designed by machine learning technologies. In recent years, the role of data science in drug discovery is evolving rapidly. We expect this evolution to continue at a rapid clip for the foreseeable future. In this talk we will discuss tools, technologies, and languages that data scientists are using to gain extra insights and value from biomedical data. The presentation will explore them in the context of three different data analytics domains - descriptive, predictive, and prescriptive.
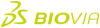
Claire Pancrace, Dr., Solution Consulting Senior Manager at BIOVIA, Dassault Systèmes
Join the presentation to see how a scientific collaborative and business platform can help design lead candidates in a integrated environment with modeling and simulation, AI and Machine Learning and laboratory informatics capabilities. Discover how BIOVIA can help reduce the number and increase the success rate of experiments by designing molecules with desired properties. Understand how to democratize high quality predictive models while maintaining data integrity and protecting your intellectual property
Session Break and Transition to Plenary Keynote16:30
ROOM LOCATION: MOA 9
PLENARY KEYNOTE PROGRAM
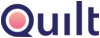
Next-generation biopharma workflows require user-driven stewardship of data that gives your enterprise custody and validation of the full "data chain of custody": from instrument, to scientist, to filing. This data chain of custody requires a flexible private-cloud storage system that integrates business documents, large instrument files, and semi-structured metadata into a single, cross-functional storage layer that meets the scale requirements of dry scientists and the usability requirements of wet scientists.
PLENARY KEYNOTE PRESENTATION: Novartis Institute for Biomedical Research Data Strategy
Philippe Marc, PhD, Executive Director and Global Head, Integrated Data Sciences, Novartis Institutes for BioMedical Research
As part of the larger enterprise digital journey, and as part of the Novartis Research Master Plan, the Novartis Institute for Biomedical Research (NIBR) defined an updated data and data management strategy. This data strategy falls into a broader digital strategy which has many additional priority areas: Information technology, Artificial Intelligence, external science, decision support for drug discovery and early development to name a few. The NIBR data management strategy is based around four pillars:
1. Data Culture: Treat data as a corporate asset
2. Data Management: Structure and link data
3. Data Science: Develop products and insights based on data
4. Data Enterprise: Lead the enterprise on data
Welcome Reception in the Exhibit Hall with Poster Viewing (Room Location: MOA 11)17:30
Close of Day18:30
Wednesday, 19 October
Registration and Morning Coffee (Foyer)08:30
ROOM LOCATION: MOA 9
PLENARY KEYNOTE PROGRAM
PLENARY KEYNOTE PRESENTATION: Digital Twins: The Virtual Future of Medicine
Peter Coveney, PhD, Professor of Physical Chemistry, Honorary Professor of Computer Science, Director of the Centre for Computational Science, University College London
The purpose of building digital twins of ourselves is to create an organizational principle for modern predictive and personalized medicine. This talk will discuss the principles on which such digital twins may be constructed and used for clinical and healthcare purposes. The roles of multiscale modelling and simulation, artificial intelligence and uncertainty quantification will be described as essential elements in the drive to making actionable predictions from digital twin simulations.
Best of Show Award Ceremony and Refreshments in the Exhibit Hall with Poster Viewing (Room Location: MOA 11)10:15
ROOM LOCATION: MOA 2
APPLICATION OF AI AND MACHINE LEARNING IN PRECLINICAL AND CLINICAL PHASES
Machine Learning Operations (MLOps) in Preclinical Research Workflows
Machine learning (ML) and AI have already been widely adopted throughout the entire preclinical drug discovery pipeline. However, without an appropriate infrastructure to manage them, most of these AI/ML models will never deliver their expected value to the organizations that adopt them. Two years ago a diverse team of scientists set out to explore the business and technical challenges and opportunities of bringing operational machine learning (aka MLOps) to pre-clinical research workflows. Fast forward to this day, the same group of people has been empowered to implement and operate a state of the art MLOps service tailored to the needs of the pRED (Pharma Research and Early Development) organization in Roche. In this session, we would like to share all exciting accomplishments but also our challenges and our learnings and in addition collect feedback and have a conversation on how the Bio-IT World sees the MLOps impact in the fields of biomedical research, drug discovery & development, and healthcare.
Why Diverse Data Matters When Using Machine Learning for Drug Discovery and Research
Maxine Mackintosh, PhD, Programme Lead - Diverse Data, Genomics England; Co-founder, One HealthTech
Studies of human genetics have largely focused on populations from WEIRD (Western, Educated, Industrialized, Rich, Democratic) countries which has resulted genomic insights that are not generalizable to all populations. Most studies, trials and papers conclude with a call to action to recruit and use more diverse genomes, and yet the proportion of non-European ancestries in genomic studies is diminishing. To address this gap, we must work across the whole pipeline of genomic research and health care delivery, from the populations we work with and the data we collect, to the analyses we carry out and the availability of genetic testing. This talk will cover the complex challenges taking an end-to-end approach to diversifying genomics involves, and what we might do to reduce bias in our data-driven systems in precision medicine.
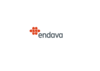
Jasmina Smailovic, PhD, Senior Data Science Consultant, Endava
Introduction of Automation improves efficiency of analysis, while enabling Scientists to be more productive and focus on what matters to them most. This talk will present how we Automate complex Workflows in the domain of Microscopy. We will discuss the typical workflow, challenges it brings, as well as our approach to tackle these challenges through application of AI and Automation. Moreover, we will show practical examples of the developed customized solutions.
Networking Lunch (Room Location: MOA 11)12:40
Dessert Break in the Exhibit Hall with Poster Viewing (Room Location: MOA 11)13:40
APPLICATION OF AI AND MACHINE LEARNING TO PRECISION DOSING AND DRUG REPURPOSING
Precision Repositioning with High-Resolution Patient Stratification: Finding New Treatments for Unmet Medical Needs
Steve Gardner, PhD, CEO and Co-Founder, PrecisionLife
Finding druggable targets for complex chronic diseases is particularly difficult using existing drug discovery approaches. This leaves significant pockets of patients with unmet medical needs, but also many opportunities to reposition active molecules originally designed to treat another disease, which act on mechanisms relevant to the needs of currently ineffectively treated patients. High-resolution patient stratification based on combinatorial analytics offers an accurate and scalable route to map effective drugs to patient subgroups across multiple new disease indications, providing a faster, cheaper, and derisked route to their approval.
Enabling Precision Dosing through Model-Based Artificial Intelligence
Benjamin Ribba, PhD, Scientist, Predictive Modeling and Data Analytics, Roche
Precision dosing, or delivering the right dose for every patient, is a key enabler of precision medicine. However, nowadays recommended dose for most of medicines is not personalized. There are many valid reasons behind the "one dose fits all" approach of today's practice for testing and finally recommending medicine dosage both during R&D (lean development) and marketed use (minimal complexity of drug usage). Exploring precision dosing in R&D is key for leveraging the full potential of a therapeutic intervention, enhancing the change of development success and providing a therapeutic benefit personalized to each patient's needs. To explore precision dosing in R&D, methodologies needs to be developed and tested, in particular, those which can take advantage of highly dimensional biomarkers characterizing today's era of digitalization. Learning from multidimensional data to generate dynamic rules is a primary benefit from reinforcement learning. In special cases such as sedation in intensive care units, reinforcement learning is applied and works by automatically adapting dosing through continuous monitoring of patient’s consciousness within a feedback loop process. We further explore the problem of optimal control of sedation with Propofol by considering inherent factors characterizing model-based drug development. In particular, the opportunity to use pharmacokinetic-pharmacodynamic (PKPD) model as a simulation engine to generate knowledge from dosing scenarios which cannot be tested experimentally; the partial knowledge of patient’s drivers of drug effect; and finally, the presence of difference level of variability in the data. Through simulation, we show that learning purely from retrospective trial data, even with a sample size up to 500 patients, gives worst precision than heuristic dosing strategies. However, embedding PKPD model of drug effect within the RL algorithm provides best accuracy. Extending the definition of reinforcement learning agent state leads to further increase accuracy of optimal dosing policy. In this study case, residual variability has a large impact on the efficiency of the algorithm. Model-based reinforcement learning constitutes a promising approach for precision dosing with a need for additional research to realize its potential for application to real data from clinical settings and drug development.
DrugX – AI-Based Drug Repurposing Pipeline
Kamal Rawal, PhD, Associate Professor, Bioinformatics & Computational Biology, Amity University
We have created one of the largest resources related to COVID-19 by compiling datasets from 50 publicly available sources [https://tinyurl.com/DrugX-pipeline]. An expert curation strategy was combined with a text mining system to screen over 552700 abstracts that are relevant to SARS CoV-2. We obtained 107 interactions amongst the SARS-CoV2 genes, 820 interactions between SARS-CoV2 genes and drug molecules, 4045 interactions between drug molecules and side effects and 8403 interactions amongst the drug molecules. Utilizing all these interactions, we constructed 5 heterogeneous networks covering the molecular pathophysiology of COVID 19. These networks include CoV-Human, CoV-CoV, drug-CoV, drug-drug, and drug-side-effect. We also obtained a scale-free multi-layer network of 189 nodes and 554 edges, composed of 20 SARS CoV-2 genes, 148 human genes, 7 drugs, and 14 side effects. Further, we deployed an automated docking pipeline to conduct large-scale docking studies (~1 million docking experiments) involving several drugs and targets to identify network-based binding propensities [Rawal et al 2022 A]. Next, we developed a multi-modal pipeline named Cov-DrugX (http://drugx.kamalrawal.in/drugx/) to repurpose drugs for COVID-19 treatment. It incorporates several independent modules used for ranking and characterizing the drugs. Each module utilizes data from diverse sources, such as the DGIdb, DrugBank, SIDER, etc. We have labeled these modules as DL-200, D-Side_Effect, D-Target, D-Gene_Expression, D-Dock, D-Circuit, D-AI_ranking, and D-Symptoms. We have incorporated a deep-learning (DL) system (multi-layered neural network) in modules such as DL-200 and graph neural network in D-AI_ranking. A new scoring mechanism is introduced to standardize heterogeneous outputs generated by different modules. We have shown applications of COV-Drug-X on COVID-19 related drugs such as molnupiravir, ritonavir, cilostazol, and rabeprazole. Further, we have conducted screening of 2700+ FDA-approved drugs and identified ramipril, imatinib, mitoxantrone, and rifampicin as the top-ranking hits. Next, we also screened large chemical and drug libraries using our platform. This exercise has revealed cyclosporine as one of the top-ranking candidates. To support our predictions, we created evidence against each top-ranking hit using the literature search [Verma and Rawal et al 2022].
Close of Conference15:45